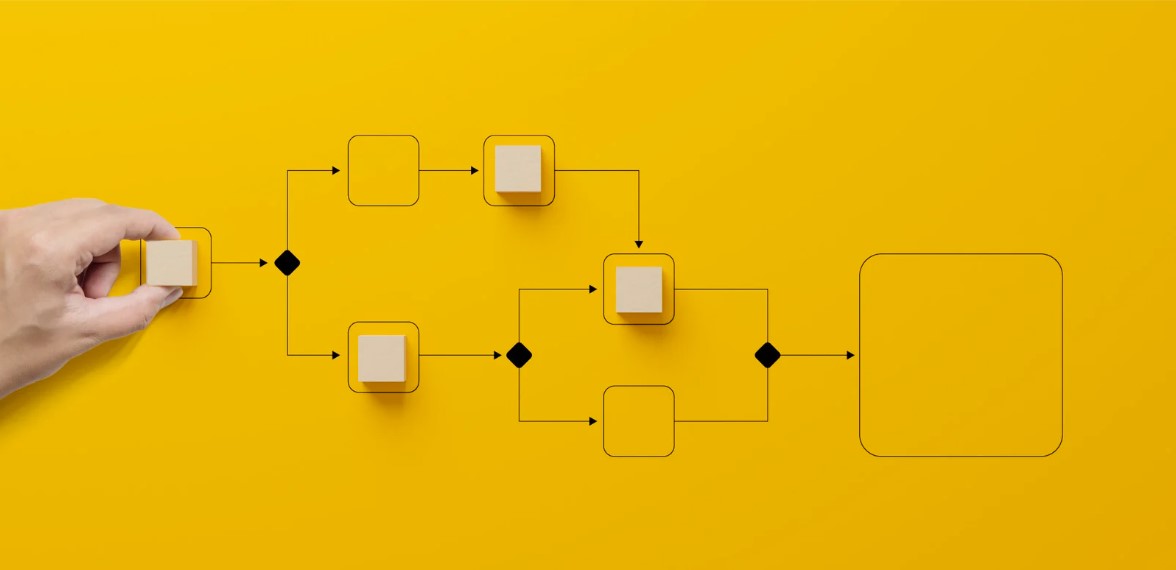
Causal Inference
Causal Inference Collaboratory
Understanding the cause-and-effect relationship between two things can help us understand the potential impact of an intervention in public health research and beyond
Causal inference helps determine when correlation might tell us about causation, which is what researchers are often interested in. The causal inference framework rigorously outlines the assumptions we need to make to draw conclusions beyond apparent associations.
Building upon the existing strengths of the college through a community of collaboration, our vision is to be a central resource for researchers interested in advancing causal inference research. By bringing together researchers with diverse expertise, our mission is to foster causal reasoning within interdisciplinary research to yield novel strategies and grant proposals. We will offer regular meetings, training sessions, and assistance in the preparation for grants that evaluate causal effects to answer research questions. Our collaborative group is designed to not only build upon the pillars of excellence of our college from a methodological standpoint but also provide hands-on experience and help with projects entailing complexities related to causality. This work will address challenges central to public health research, which are relevant both for randomized and observational studies across the college and university.
Collaboratory Goals
Goal 1
Goal 1: Conduct a comprehensive review of how causal theory and methods can provide innovative insights into public health research. We want to share the results and align this research with the primary goals of the College of Public Health, which includes exploring how causal techniques can enrich the goals of comparative effectiveness to inform healthcare.
Goal 2
Goal 2: Provide causal inference training by conducting workshops and collaborative projects. We will organize a series of workshops that provide hands-on experience with various research methods for any interested individuals. This will equip participants with the necessary skills to conduct their own research and contribute to ongoing causal projects.
Goal 3
Goal 3: Create a platform for collaboration and continuous learning via working groups and small causal conferences. Faculty and students will meet for journal club discussions, present ongoing research, and brainstorm aims for grant submissions. A primary focus of this component is to collaborate on competitive grants related to causal inference research.
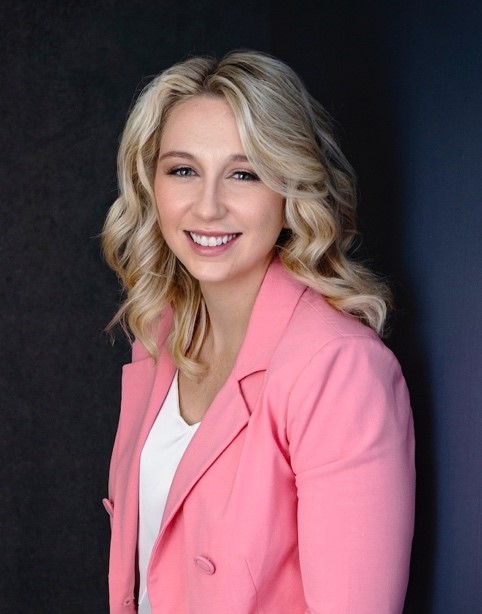
Dr. Emily Roberts, Team Lead
Department of Biostatistics
Dr. Roberts has experience utilizing and developing causal inference techniques, particularly principal stratification methodology and Bayesian estimation for surrogate endpoint validation. Many of these projects employ causal inference methods such as propensity score weighting, mediation analyses, and adjustment for selection and confounding bias in complex survey designs and data collection.
Collaborators
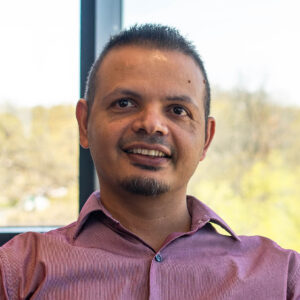
Hari Sharma
Department of Health Management and Policy
Dr. Sharma’s research centers on critical issues related to the financing, workforce, and disparities in access and quality of post-acute and long-term care in the US, with a particular focus on nursing homes. He uses quasi-experimental methods to estimate the causal effect of policies on different outcomes. Recent projects include evaluating supplemental payments to nursing homes, assessing the impact of rural nursing home closures, and documenting occupational injuries in US nursing homes.
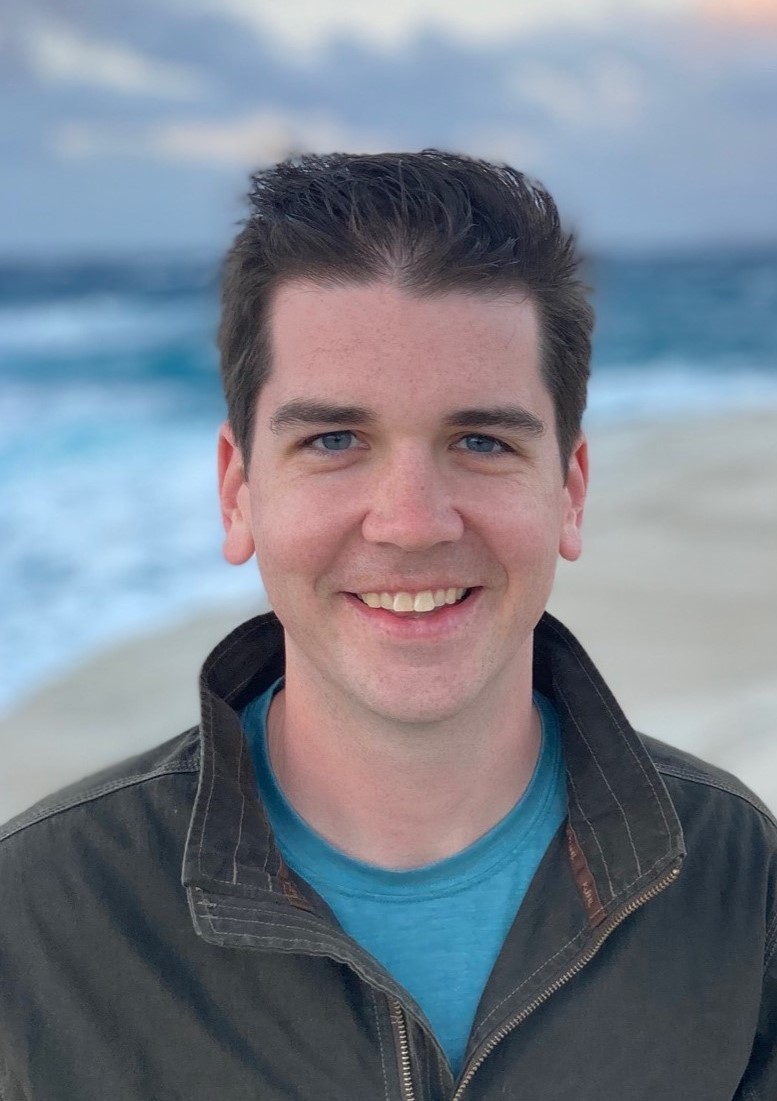
Nathan Wikle
Department of Statistics
Dr. Wikle has worked on developing causal methods for environmental health data analysis. His research includes the estimation of causal effects in settings with spatial interference. His current work includes the estimation of causal effects in the presence of non-local confounding, as well as developing new methods for causal inference with spatio-temporal data under stochastic interventions.
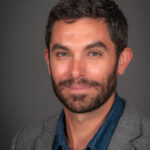
Jonathan Platt
Department of Epidemiology
Dr. Platt has experience studying the epidemiology of adolescent psychiatric disorders and suicide. His methodological expertise has focused on estimating developmental and social mechanisms underlying psychiatric disorders using causal inference approaches, as well as modeling spatial and temporal effects and the use of machine learning methods to identify novel health risk patterns.
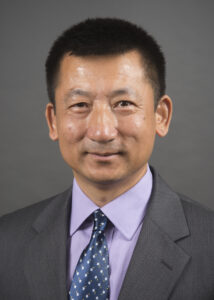
Kai Wang
Department of Biostatistics
Dr. Wang has conducted some theoretical work on mediation analysis. He compared the full-information maximum likelihood estimate and the iterative feasible generalized least squares estimate in terms of their variance matrices for a mediation model that contains a treatment-mediator interaction term. His current research focuses on theoretical aspects of Mendelian randomization analysis.
Collaboratory Meetings
End of Semester Event
May 7, 2025, 11 am to 2 pm
11-11:15 Causal Inference Collaboratory Overview, Accomplishments, Next StepsĀ (View PowerPoint)
11:15-12:15 Speed Presentations on Causal Inference Research
Targeted estimation of the effects of childhood adversity on fluid intelligence in a US population sample of adolescents
Effect of Paid Sick Leave on Child Health
Valid inference for two sample summary data Mendelian randomization
Xin Zan’s multi-topic overview
Making Medicaid Work
Causal Inference and Combining Sources of Evidence in Diabetes Studies
12:15-12:30 Break/lunch is served
12:30-1:20 Presentation and full group brainstorming
1:30-2:00 Small group grant brainstorming
Spring 2025
February 4 at 11:30 a.m.
February 17 at 12:30 p.m.
March 11 at 11:30 a.m.
March 24 at 12:30 p.m.
April 8 at 11:30 p.m.
April 22 at 2:30 p.m.
Fall 2024
September 11 at 2:30 p.m.
September 23 at 12:30 p.m.
October 9 & 23 at 2:30 p.m.
November 6 at 2:00 p.m.
November 20 at 2:30 p.m.
December 4 & 18 at 2:30 p.m.
Meetings and workshops covered areas such as:
- Causal mediation
- Mendelian randomization and instrumental variables
- Causal inference with environmental exposures, interference, and applications for spatial data
- Causal inference techniques and clinical trial design and analysis
- Use of surrogate markers, managing treatment non-adherence, and combining randomized and observational data
- Practical introduction to Monte Carlo simulation studies
- Statistical intuition underlying causal identification
- Plan, conduct, and interpret simulation studies, particularly through the lens of sensitivity to causal assumptions
Our main goal is to expand our team and bring together researchers interested in causal inference via the Collaboratory! Please contact Lila Basnet to be added to the calendar invites and attend our sessions to get involved. Reach out to Emily Roberts with questions.
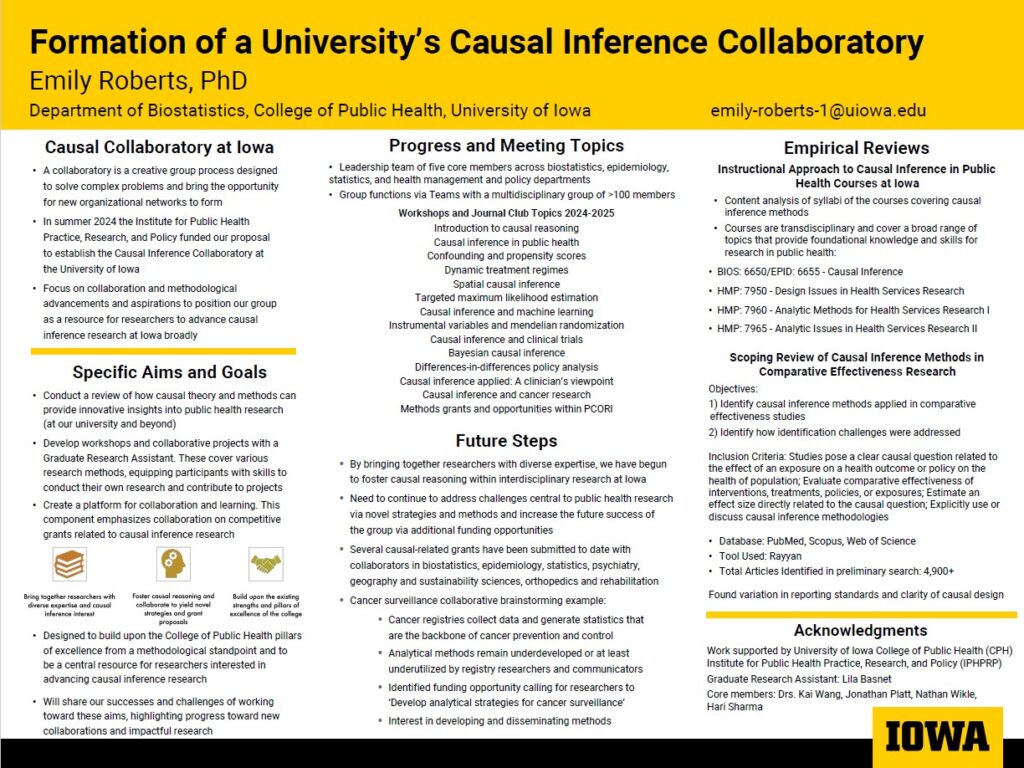
Resources
In fall 2024, our group discussed the important role of causal inference in public health. In the paper, the authors discuss the history of frameworks in approaching causal inference over time. Importantly, they advocate for the teaching and use of the modern potential outcomes framework for evaluating causality.